Predictive Analytics as Part of Business Intelligence
Essentially, predictive analytics involves determining historical data using algorithms and statistical models, analyzing machine-learning approaches – a subdiscipline of artificial intelligence – and predicting the probabilities of future developments. In contrast to data analytics, it is not a matter of looking at the here and now and how you got there, but rather a matter of assessing future-oriented trends and developments and determining them with a high degree of precision.
The principle is basically not new. Weather forecasts have been made in a similar way for some time now. The risk management approaches of business models in financial services are also often based on predictive analytics methods, for example to determine how likely a customer is to default.
What is now new, however, is the easily accessible, intuitive, and inexpensive technology that no longer makes these approaches available only to statisticians and mathematicians. On the one hand, it also enables them to find their way into company management and, on the other hand, it produces increasingly accurate forecasts. Among the main drivers of predictive analytics are big data or the mass collection and evaluation of data, faster and cheaper computer technology, and intuitive and very powerful software, which takes over data collection and preparation, as well as the analysis and complex modelling for the user in large parts.
The Process behind Predictive Analytics
The process steps on which this technology is based can be simplified as follows:
-
- Definition of the project and clarification of the goal: What is the desired result of the analysis and what questions should be answered? On what data basis can this analysis be carried out?
- Data collection: Data is collected from various sources, processed, and prepared for analysis.
- Data analysis: Valuable information is obtained from the data.
- Statistics: The use of standard statistical models makes it possible to test assumptions and hypotheses.
- Modelling: Development of a model to determine probable future events based on automated decisions of the model.
- Deployment: Use of the model to make business decisions.
- Monitoring: The results of the models are compared with actual events to monitor their accuracy.
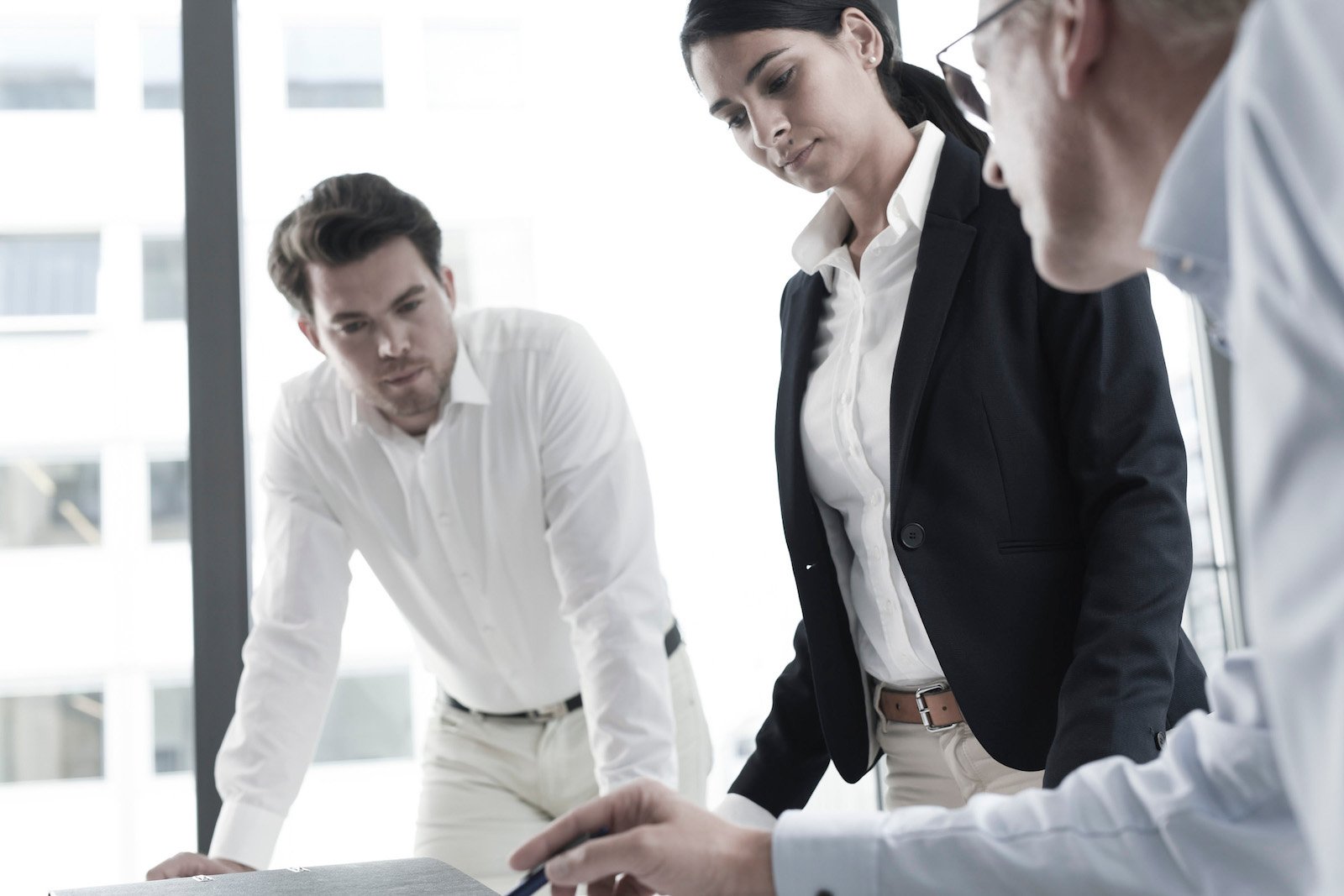
Application Examples of Predictive Analytics
The field of application of predictive analytics approaches is now broad. In any area where sufficient data is available, the technology can basically be applied.
Liquidity Planning Using Predictive Analytics
Good liquidity management aims to always keep the right amount of liquid funds in the company – on the basis of historical data on the payment behavior of existing customers, supplemented by external data if necessary. This could be public holiday data or credit scoring of debtors, for example. Future incoming payments can be forecast very accurately. Any liquidity bottlenecks can thus be detected at an early stage and mitigated in good time.
Sales and Marketing Planning Based on Predictive Analytics
Analogous to the payment behavior of customers, their purchasing behavior can of course also be analyzed in a similar way in order to obtain statements on future sales development. On this basis, delivery bottlenecks can be avoided and sales slumps can be corrected in good time. This analysis of behavior patterns can be even more far-reaching, predicting events such as house building or an upcoming wedding based on certain account movements.
Predictive Analytics for Production Planning
Complex modelling allows orders that have not yet been realized to be taken into account in production planning and control, so that there is no need to completely reschedule if the order situation changes. Predictive analytics can also be used to design the maintenance of machines and systems with foresight and to link it to the automatic replenishment of spare parts so that downtimes do not occur.
All these examples illustrate that predictive analytics approaches can create an immense competitive advantage in companies in any industry and are not limited to just a few subareas. The times of gut feeling and expert assessment as the means of choice in corporate planning are slowly but surely coming to an end. It gives way to the always-accurate results of data-based models and algorithms.
Would you like to find out how data analysis approaches, and predictive analytics in particular, can generate real added value in your company? Talk to us. We look forward to hearing from you.